

Here, we introduce a new, potentially widely applicable and accurate drug target identification strategy based on network analysis to identify the interactions of active components in TCM formula and target proteins. Thus apart from target identification of a bioactive compound, this network analysis method is more beneficial in identifying unknown targets of active compounds in TCM formula simultaneously in an unbiased fashion. In addition, metabolomics and network pharmacology employed global profiling methods for the comprehensive analysis of altered metabolites and target proteins, providing insights into the global state of entire organisms, which are well coincident with the integrity and systemic feature of TCM formula. As a combination of approaches is most likely to bear fruit, the combination of network pharmacology and metabolomics called network analysis could increase the degree of accuracy of target identification of network pharmacology. Once we use network pharmacology to predict potential targets of active components in Traditional Chinese Medicine (TCM) formula 13, a component-target protein-related protein-metabolite network can be constructed with the combination of network pharmacology and metabolomics. With the advent of the era of big data, now there are large amounts of data about known and predicted protein interactions 12. Therefore using metabolomics for target identification run into the bottleneck.Īs bioactive molecules exert their effects through direct physical association with one or more cellular proteins 1, these target proteins will then act on related proteins, above proteins eventually affect the content of related metabolites. Whereas metabolomics has been mainly developed to identify drug(s)-affected pathways 10, 11, the “readout”, such as proteins in the pathway, is often far downstream from the drug targets.
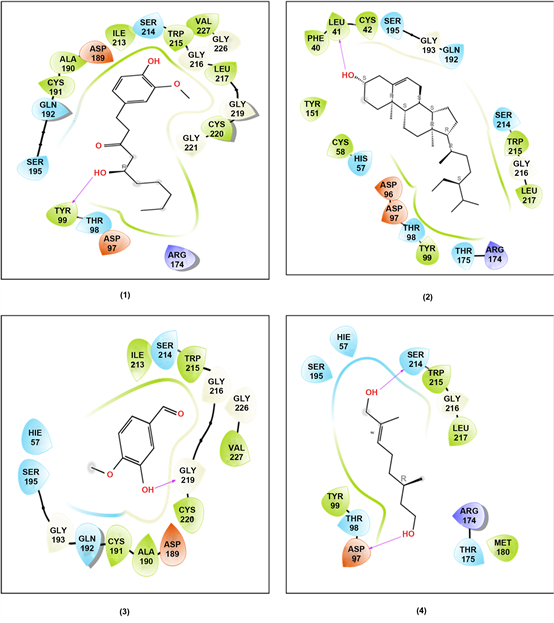
These two methods have been proved successful in target identification of both many compounds and one drug 6, 7, 8, 9.

A proteomic or transcriptomics approach for identification of binding proteins for a given small molecule or compounds in herbs involves comparison of the protein expression profiles for a given cell or tissue in the presence or absence of the given molecule(s). With the indirect approach, such as system biology approaches, including proteomics, transcriptomics and metabolomics, are the major tools for target identification and have an unbiased attitude towards all active compounds 5. Moreover, because of above characteristics, this approach is only suitable to identify targets of one drug once and cannot afford target identification of many compounds simultaneously, such as active components in herbs. Many compounds cannot be modified without loss of binding specificity or affinity 4. The direct approach utilizes affinity chromatography often with compound-immobilized beads. There are two fundamentally different approaches to identify molecular targets of bioactive molecules: direct and indirect 3. The detection of drug-target interactions is therefore necessary for the characterization of compound mechanism of action 2. We envisage that network analysis will also be useful in target identification of a bioactive compound.īioactive compounds exert their biological activities through direct physical binding to one or more cellular proteins 1. Results indicated that hypaconitine, mesaconitine, higenamine and quercetin in SND can directly bind to TNF-α, reduce the TNF-α-mediated cytotoxicity on L929 cells and exert anti-myocardial cell apoptosis effects. Among the 25 targets predicted by network analysis, tumor necrosis factor α (TNF-α) was firstly experimentally validated in molecular and cellular level. At last, network analysis was conducted to identify most possible targets of components in SND. The key enriched processes, pathways and related diseases of these target proteins were analyzed by STRING database. Then, we employed network pharmacology including text mining and molecular docking to identify the potential targets of these components. To begin with, 48 potential active components in SND against heart failure were predicted by serum pharmacochemistry, text mining and similarity match. In this study, we firstly proposed network analysis by integrating data from network pharmacology and metabolomics to identify targets of active components in sini decoction (SND) simultaneously against heart failure. Identifying the molecular targets for the beneficial effects of active small-molecule compounds simultaneously is an important and currently unmet challenge.
